Гости подкаста — специалисты в разных сферах AI. С ними мы обсуждаем профессию AI Researcher, карьерный рост и собеседования, а также исследования в разных областях, от фундаментального AI до медицины и квантовых компьютеров.
…
continue reading
Most learning is superficial and fades quickly. This podcast will equip you to move to learning that is durable because it is deep. Deep learning lasts because it respects the way the brain works. Inquiring minds want to know "how" and "why"—not just what!
…
continue reading
PolyAI's CEO/co-founder Nikola Mrkšić and team invite guests to candidly discuss trends and tech in AI, voice throughout the enterprise, and nailing the customer experience.
…
continue reading
Individual topics and concepts from AI ML DL made simple using Notebook LM. From Brian Carter. https://keynotespeakerbrian.com/
…
continue reading
Long-form technical deep dives with Andrej Karpathy and Justin Johnson
…
continue reading
Welcome to The Deep Learning Crowd Podcast. We talk about individual journey's in the world of AI and discuss topics around Deep Learning. We discover first hand from our guests about some of the most interesting applications their companies are using right now.
…
continue reading
Find me on Github/Twitter/Kaggle @SamDeepLearning. Find me on LinkedIn @SamPutnam. This Podcast is supported by Enterprise Deep Learning | Cambridge/Boston | New York City | Hanover, NH | http://www.EnterpriseDeepLearning.com. Contact: Sam@EDeepLearning.com, 802-299-1240, P.O. Box 863, Hanover, NH, USA, 03755. We move deep learning to production. I teach the worldwide Deploying Deep Learning Masterclass at http://www.DeepLearningConf.com in NYC regularly and am a Deep Learning Consultant ser ...
…
continue reading
Most AI research today is done by elite universities and corporate labs. The pursuit of science and creativity should be a viable project for any person, from any walk of life, who is excited by that feeling of mystery and building something that grows. chloe is an end to end neural network chatbot written in PyTorch based on the transformer. Accomplishing goals through conversation is a task we can relate to, chatbots are an ideal agent through which to connect new research to our current u ...
…
continue reading
Send us a text Bonus breaking news episode of the Deep Learning with PolyAI podcast! Hosts Damien and Nikola chat about the recent release of DeepSeek's open-source R1 model, which has sparked widespread reaction across the tech world. The R1 model, created with limited resources, has matched or exceeded benchmarks of leading models from OpenAI and…
…
continue reading
Most schools design curriculum around a fact forward approach. Facts are always in the foreground while ideas lurk in the background and generally make only cameo appearances. This is exactly wrong. The role of facts is to support ideas. Facts are organized by ideas and not the reverse. It is ideas that have consequences. While there may be "inconv…
…
continue reading
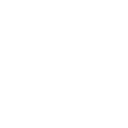
1
Соревнования по машинному обучению: взгляд со стороны участника и организатора
1:21:24
1:21:24
Відтворити пізніше
Відтворити пізніше
Списки
Подобається
Подобається
1:21:24
Это подкаст, который мы записали в рамках нашего сотрудничества с ecom.tech. И гости этого выпуска — Шарафетдинов Ринат и Евгений Финогеев. Давайте узнаем про то, как набраться сил поучаствовать в своем первом хакатоне с одной стороны, а с другой разберемся в том, зачем такие соревнования нужны компаниям-заказчикам и организаторам. На подкасте мы, …
…
continue reading
Send us a text Kylie takes the helm for this episode of Deep Learning with Poly AI, where she and PolyAI CEO Nikola Mrkšić chat(no GPT) about OpenAI's latest innovation: the Operator agent. From the concept of AI performing tasks such as ordering groceries, booking travel, and making restaurant reservations, to live demos of agentic requests, Kylie…
…
continue reading
Send us a text Damien and Nikola (CEO & Co-Founder of PolyAI) talk about the recently announced AI Opportunities Action Plan. The conversation highlights the UK’s historical contributions to AI, the challenges it faces due to American tech dominance, and the strategic moves to build a competitive AI ecosystem. They touch on the importance of infras…
…
continue reading
Children are all born learners—at least until they go to school. Many children and their parents are frustrated and mystified by the setbacks that are experienced at school and it doesn't need to be this way! This is the first episode of an entire season dealing with why your child may not like school and what to do about it. The season is structur…
…
continue reading
Send us a text In this episode of 'Deep Learning with PolyAI,' Damien and Nikola reflect on the significant trends in AI throughout 2024, including the rise of generative AI, the stabilization of models, and increased enterprise adoption. They also discuss the importance of customer experience and data management in AI applications. As they look fo…
…
continue reading
Send us a text Orgs establishing AI competency centers or hiring Chief AI Officers are heavy in 2025 predictions. Sit beside the fire with Damien and Nikola Mrkšić, CEO and co-founder of PolyAI, in this enlightening episode of Deep Learning with PolyAI, as we tap into the emergence of Chief AI Officers (CAIOs) in organizations, their responsibiliti…
…
continue reading
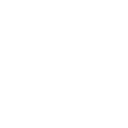
1
Детекция сгенерированных текстов и и трудности жизни AI реcерчера с Лаидой Кушнаревой
1:36:22
1:36:22
Відтворити пізніше
Відтворити пізніше
Списки
Подобається
Подобається
1:36:22
Гость выпуска — Лаида Кушнарева, выпускница мехмата МГУ, старший академический консультант в компании Хуавей и автор телеграмм канала "Техножрица". Обсудили с Лаидой: Путь Лаиды в рисерче; Задачу fake text detection: почему она сложная, что там интересного, как сейчас обстоят дела, и что насчет будущего этой задачи; Устройство процесса ревью и прин…
…
continue reading
Send us a text Time to decode the buzzterm du jour - in this episode of Deep Learning, Damien and Nikola discuss the emerging (or is it?) concept of agentic AI, and how AI systems that act autonomously and perform complex tasks could transform individual and corporate life. From planning vacations to automating business processes, we talk about the…
…
continue reading
Send us a text In this episode of 'Deep Learning with PolyAI,' host Damien and guest Kylie Whitehead, Senior Director of Brand and Marketing at PolyAI, discuss the evolving expectations of consumers when it comes to customer service, especially in the context of automation. Drawing from key industry survey results, they explore how organizations ca…
…
continue reading
Worldview is the key grid through which we filter and formulate ideas, yet it is not systematically developed in most educational programs. This is particularly likely on the university level. Make no mistake—a worldview is being developed anyway, but likely full of flaws and non sequiturs.Michael Gray
…
continue reading
Send us a text ** Catch a replay of VOX here: https://poly.ai/vox-2024/ ** Damien and PolyAI CEO Nikola Mrkšić as they rewind to Thursday, November 14th, covering the evolution and challenges of automatic speech recognition in voice AI and automation for contact centers, emerging trends in CX and customer satisfaction, and tips on boosting ops with…
…
continue reading
Introducing a novel transformer architecture, Differential Transformer, designed to improve the performance of large language models. The key innovation lies in its differential attention mechanism, which calculates attention scores as the difference between two separate softmax attention maps. This subtraction effectively cancels out irrelevant co…
…
continue reading
No one wants to kill the joy of learning in a young child, but that's likely with the majority of preschool and elementary pedagogies. This podcast helps you sort through the educational philosophy underlying some major options.Michael Gray
…
continue reading
Introducing, ScienceAgentBench, a new benchmark for evaluating language agents designed to automate scientific discovery. The benchmark comprises 102 tasks extracted from 44 peer-reviewed publications across four disciplines, encompassing essential tasks in a data-driven scientific workflow such as model development, data analysis, and visualizatio…
…
continue reading
Both sources explain neural network pruning techniques in PyTorch. The first source, "How to Prune Neural Networks with PyTorch," provides a general overview of the pruning concept and its various methods, along with practical examples of how to implement different pruning techniques using PyTorch's built-in functions. The second source, "Pruning T…
…
continue reading
The source is a chapter from the book "Dive into Deep Learning" that explores the historical development of deep convolutional neural networks (CNNs), focusing on the foundational AlexNet architecture. The authors explain the challenges faced in training CNNs before the advent of AlexNet, including limited computing power, small datasets, and lack …
…
continue reading
This text is an excerpt from the "Dive into Deep Learning" book, specifically focusing on the processing of sequential data. The authors introduce the challenges of working with data that occurs in a specific order, like time series or text, and how these sequences cannot be treated as independent observations. They delve into autoregressive models…
…
continue reading
This excerpt from "Mental Models," a chapter in the "People + AI Guidebook," focuses on the importance of understanding and managing user mental models when designing AI-powered products. The authors discuss how to set expectations for adaptation, onboard users in stages, plan for co-learning, and account for user expectations of human-like interac…
…
continue reading
This excerpt from Hugging Face's NLP course provides a comprehensive overview of tokenization techniques used in natural language processing. Tokenizers are essential tools for transforming raw text into numerical data that machine learning models can understand. The text explores various tokenization methods, including word-based, character-based,…
…
continue reading
This research paper examines the efficiency of two popular deep learning libraries, TensorFlow and PyTorch, in developing convolutional neural networks. The authors aim to determine if the choice of library impacts the overall performance of the system during training and design. They evaluate both libraries using six criteria: user-friendliness, a…
…
continue reading
This document provides a comprehensive set of rules for building and deploying machine learning systems, focusing on best practices gleaned from Google’s extensive experience. The document is divided into sections that cover the key stages of the machine learning process, including launching a product without ML, designing and implementing metrics,…
…
continue reading
The research paper "MoE-Mamba: Efficient Selective State Space Models with Mixture of Experts" explores a novel approach to language modeling by combining State Space Models (SSMs), which offer linear-time inference and strong performance in long-context tasks, with Mixture of Experts (MoE), a technique that scales model parameters while minimizing…
…
continue reading
Send us a text Damien and Nikola talk rising aggression towards contact center agents and the protective role of AI automation. Highlighting alarming stats like 65% of people abandoning brands after one bad experience and a 90% increase in customer service abuse during peak seasons, they address the issue and how AI can deflect initial customer rag…
…
continue reading
We discuss how to build Agentic Retrieval Augmented Generation (RAG) systems, which use AI agents to retrieve information from various sources to answer user queries. The author details the challenges he faced when building an Agentic RAG system to answer customer support questions, and provides insights into techniques like prompt engineering and …
…
continue reading
Let's get RE(a)L, U! This research paper explores the impact of different activation functions, specifically ReLU and L-ReLU, on the performance of deep learning models. The authors investigate how the choice of activation function, along with factors like the number of parameters and the shape of the model architecture, influence model accuracy ac…
…
continue reading
Attempting to read the Bible through each year is a source of frustration and guilt to many as they repeatedly fall behind their reading schedule. Is this yearly ritual a spiritual discipline that advances discipleship or does it substitute a false sense of breadth for real depth?Michael Gray
…
continue reading
This lecture from Stanford University's CS229 course, "Machine Learning," focuses on the theory and practice of linear regression and gradient descent, two fundamental machine learning algorithms. The lecture begins by motivating linear regression as a simple supervised learning algorithm for regression problems where the goal is to predict a conti…
…
continue reading
This video discusses the vanishing gradient problem, a significant challenge in training deep neural networks. The speaker explains how, as a neural network becomes deeper, gradients—measures of how changes in network parameters affect the loss function—can decrease exponentially, leading to a situation where early layers of the network are effecti…
…
continue reading
A scientific paper exploring the development and evaluation of language agents for automating data-driven scientific discovery. The authors introduce a new benchmark called ScienceAgentBench, which consists of 102 diverse tasks extracted from peer-reviewed publications across four disciplines: Bioinformatics, Computational Chemistry, Geographical I…
…
continue reading
We discuss how to utilize the processing power of Graphics Processing Units (GPUs) to speed up deep learning calculations, particularly in the context of training neural networks. It outlines how to assign data to different GPUs to minimize data transfer times, a crucial aspect of performance optimization. The text highlights the importance of unde…
…
continue reading
This paper provides a comprehensive overview of deep generative models (DGMs) and their applications within transportation research. It begins by outlining the fundamental principles and concepts of DGMs, focusing on various model types such as Variational Autoencoders (VAEs), Generative Adversarial Networks (GANs), Normalizing Flows, and Diffusion…
…
continue reading
This research paper presents the development and evaluation of an AI-driven Smart Video Solution (SVS) designed to enhance community safety. The SVS utilizes existing CCTV infrastructure and leverages recent advancements in AI for anomaly detection, leveraging pose-based data to ensure privacy. The system provides real-time alerts to stakeholders t…
…
continue reading
The book titled "Mathematics for Machine Learning" explains various mathematical concepts that are essential for understanding machine learning algorithms, including linear algebra, analytic geometry, vector calculus, and probability. It also discusses topics such as model selection, parameter estimation, dimensionality reduction, and classificatio…
…
continue reading
Here we discuss three different papers (see links below) on using D-CNNs to detect breast cancer. The first source details the development and evaluation of HIPPO, a novel explainable AI method that enhances the interpretability and trustworthiness of ABMIL models in computational pathology. HIPPO aims to address the challenges of opaque decision-m…
…
continue reading
This LessWrong post explores various methods to enhance human intelligence, aiming to create individuals with significantly higher cognitive abilities than the current population. The author, TsviBT, proposes numerous approaches ranging from gene editing to brain-computer interfaces and brain emulation, discussing their potential benefits and drawb…
…
continue reading
The first source is a blog post by Max Mynter, a machine learning engineer, outlining a five-to-seven step roadmap for becoming a machine learning engineer. The post emphasizes the importance of both software engineering and data science skills alongside mathematics and domain knowledge. It then offers concrete resources, including courses and book…
…
continue reading
We discusses the importance of generalization in classification, where the goal is to train a model that can accurately predict labels for previously unseen data. The text first explores the role of test sets in evaluating model performance, emphasizing the need to use them sparingly and cautiously to avoid overfitting. It then introduces the conce…
…
continue reading
Recognizing laughter in audio is actually a very difficult ML problem, filled with failure. Much like most comedians' jokes. Let's hope some good stuff survives. This is a review of a student's final year project for a University of Edinburgh computer science course. The project focused on creating a machine learning model to detect laughter in vid…
…
continue reading
Send us a text In this episode of Deep Learning with PolyAI, join Damien and Nikola as they kibbitz over the immense energy demands of modern data centers powering advanced AI models. They explore how tech giants like Microsoft and Google are turning to nuclear energy, including the potential of fusion power, to meet these needs. From comparing the…
…
continue reading
Solving an impossible mystery... forget what you thought was possible! This is a discussion of a video from Stanford's CS224W course which focuses on the many applications of graph machine learning, a field that utilizes graph data structures to solve complex problems. The speaker highlights different tasks and their associated applications, classi…
…
continue reading
A research team from EyeLevel.ai has found that vector databases, which are commonly used in RAG (Retrieval-Augmented Generation) systems, have a scaling problem. Their research shows that the accuracy of vector similarity search degrades significantly as the number of pages in the database increases, leading to a substantial performance hit. This …
…
continue reading
Probability and statistics are fundamental components of machine learning (ML) and deep learning (DL) because they provide the mathematical framework for understanding and analyzing data, which is crucial for making predictions and decisions. This excerpt from the "Dive into Deep Learning" documentation explains the essential concepts of probabilit…
…
continue reading
This research paper examines a new deep-learning approach to optimizing weather forecasts by adjusting initial conditions. The authors test their method on the 2021 Pacific Northwest heatwave, finding that small changes to initial conditions can significantly improve the accuracy of 10-day forecasts using both the GraphCast and Pangu-Weather deep-l…
…
continue reading
An introduction to the fundamental concepts of calculus, explaining how they are essential for understanding deep learning. It begins by illustrating the concept of a limit using the calculation of a circle's area, before introducing the concept of a derivative, which describes a function's rate of change. It then extends these concepts to multivar…
…
continue reading
The source, "Generative AI's Act o1: The Reasoning Era Begins | Sequoia Capital," discusses the evolution of AI models from simply mimicking patterns to engaging in more deliberate reasoning. The authors argue that the next frontier in AI is the development of "System 2" thinking, where models can reason through complex problems and make decisions …
…
continue reading
Swarm is an experimental, educational framework from OpenAI that explores ergonomic interfaces for multi-agent systems. It is not intended for production use, but serves as a learning tool for developers interested in multi-agent orchestration. Swarm uses two main concepts: Agents and handoffs. Agents are entities that encapsulate instructions and …
…
continue reading
The provided sources detail the groundbreaking work of three scientists who were awarded the 2024 Nobel Prize in Chemistry for their contributions to protein structure prediction using artificial intelligence. David Baker, a biochemist, developed a computer program to create entirely new proteins, while Demis Hassabis and John Jumper, from Google D…
…
continue reading
Dario Amodei, CEO of Anthropic, argues that powerful AI could revolutionize various fields, including healthcare, neuroscience, economics, and governance, within 5-10 years. He envisions a future where AI could cure most diseases, eradicate poverty, and even promote democracy. However, this optimistic vision is met with skepticism from Reddit users…
…
continue reading